The artificial intelligence (AI) market is set to surpass $1.4 billion by 2029, at a CAGR of 20.1 percent, according to Fortune Business Insights. This will be brought about by growing demand and applications in industries such as aerospace, healthcare, manufacturing, and automotive, as well as verticals including retail, BFSI, healthcare, food and beverages, and logistics.
“AI should not be just for business, but it has the potential to improve all of our lives,” says Grant Case, Regional Vice President, Head of Sales Engineering – APJ of Dataiku, in a TechNode Global Q&A. In this interview, he further shares how AI is fast becoming a mainstream technology for businesses. “AI allows organizations to approach complex decision-making systematically and apply judgments at scale.”
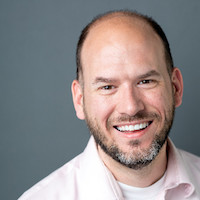
What are the three key challenges being addressed by leveraging AI?
AI is pervasive and has changed consumer lives much more than business ones so far. It helps manage our finances, recommend content we watch, choose restaurants for us, and much more.
At Dataiku, we believe this fundamental change is upon us in business, which we define as Everyday AI. Everyday AI makes the use of data almost pedestrian, with AI being so intertwined with the day-to-day that it’s part of the business (not only being used or developed by one central team). With that, I see AI broadly addressing the following challenges:
Democratization of knowledge
The world is shrinking and getting more complex, and the skills necessary to compete continue to increase. World-class organizations must bring the right set of skills to address this. Encapsulating domain knowledge in AI models allows for more individuals to apply the best practices of the best domain experts. We see this play out in specific circumstances, such as recommendation systems.
What product to offer a client, at what time, and through which distribution channels are common dilemmas for financial advisors today. With AI, advisors gain access to the best set of insights available based on the client’s needs and desired risk level. Over time, advisors learn the nuances of the model and can help further adapt to their own needs. Instead of a model doing nearly all the work, the advisor uses it more for human-in-the-loop decision-making or aided insight.
Aided insight
While there is a hunger for knowledge and understanding, organizations need to increase the effectiveness and value of their existing assets and staff. AI can be a revolution. For example, the seismic interpretation of subsurface data is vital in the development of future energy fields. Until recently, it has been a fairly intensive process requiring the input of geoscientists for extended periods.
Today, energy producers are training AI models on subsurface data to assist in the interpretation of seismic data. These powerful AI models assist geoscientists in focusing on the most relevant findings and aspects of the subsurface. What once was a multi-month process compresses to 2 – 3 days, allowing the geoscientists to spend more time running more scenarios to improve quality control and outcomes.
Systemization
A common theme among my clients is the need to make processes repeatable. AI allows organizations to approach complex decision-making systematically and apply judgments at scale. AI generates the most value for an organization when deployed in a business setting, as close to a client interaction as possible.
A great example of this is a large B2B e-commerce retailer building and training models on behalf of their clients today. This retailer trains tens of thousands of models on a monthly basis to ensure that their clients get the best recommendations when moving through the site. You cannot train 10,000+ models in isolation nor have an individual build a rule set for every client without doing it through an established process.
In terms of digital adoption or transformation, how can the more traditional enterprises accelerate their efforts in leveraging data and AI?
A traditional organization is not necessarily one that is behind in leveraging AI. Some of the most sophisticated users of AI are found within traditional industries, like manufacturing and healthcare. Just because a company is not a software firm does not mean it has to lag in using AI.
Here are three measures companies can adopt early in order to advance their AI plans and maturity.
1. AI is for all leaders, dedicate a budget
Budget is required to get started. Emphasize that AI involves the entire business, not just an innovation group, to gain organization-wide support.
2. Start small, plan big
Think through the top three to five workable use cases that can create the greatest value quickly—ideally within the first year. Projects that organizations can systemize and place into production generate the most value for the firm, so focus on them. Once AI proves its value, be ready with a portfolio of projects varying in risk and reward to keep momentum going.
3. Measure value creation from the start, emphasize AI is about innovation and survival
Be ready to move AI into the mainstream business as quickly as possible. What once was a luxury, AI is quickly becoming a necessity for businesses. However, AI cannot be any less accountable than any other set of projects. Show accountability to achieve the best ROI, then get additional funds based on potential and realized value.
Can you provide use cases or tangible values that enterprises can benefit from an AI strategy?
The number and quality of use cases that benefit from AI are substantial. However, I also like to understand where business focus is currently. We recently completed a brief with IDC focusing on Asian business use of AI, Artificial Intelligence Practices Driving Business Differentiation in the Digital-First Economy.
A major change from the 2021 InfoBrief is a transition among Asian organizations from internal use cases for AI initiatives to those that are external facing. Top adoption drivers for firms have shifted from employee productivity and improving business agility (2021) to driving product and service differentiation and customer experience (2022). This pivot occurs when organizations mature in their AI journey.
We see many use cases and much of the value of AI originating in interactions with a customer. An example would be a large pharmaceutical company using AI to segment and engage with healthcare providers based on a host of factors including location, practice, historic interactions, current research interest, and others helping to drive $10+ million dollars in new revenues.
I recommend clients sit down as a cross-functional team and list initiatives that have friction points with their clients. Friction points are one of the best places to find potential and highly valuable use cases. This includes forecasting customer demand, identifying products of interest to recommend to clients, interpreting the voice of customer information at scale, managing customer bookings, even optimizing F&B spend for conventions and retreats.
These are great starting points, but the list continues across all industries and all functions. In 3 – 5 years, AI will affect every part of every organization.
Kindly share success stories or case studies involving enterprise use of AI.
Here are some examples of the power of AI, one in the business space and one I consider to be AI for good.
GE Aviation found early on in their AI journey that there were not enough data scientists and machine learning engineers to address every one of their potential use cases. They turned to Dataiku to enable a digital workforce to do a great deal of their own analysis and machine learning work, while also allowing their specialists to focus on moonshot projects and continue to oversee the development of projects by those non-DS employees.
It was translated to GE Aviation engineers using Dataiku to collaborate and build a deep learning model on its previous piston designs. Whereas before this was done through computational fluid dynamics simulations taking place over two days, the new AI model allowed the team to evaluate millions of design changes in 15 mins. This and other AI projects of the same nature have cut GE’s design times in half.
AI should not be just for business, but it has the potential to improve all of our lives. Dataiku, through its ikig.ai initiative, has been a long-term partner of The Ocean Cleanup. Last month, we were thrilled to hear the announcement that The Ocean Cleanup had removed its first 100,000 KG of rubbish from the Great Pacific Garbage Patch. The organization uses Dataiku and AI to monitor and forecast movement of the pile, understand the different elements, and observe and analyze the performance of System 002, the structure responsible for the harvesting of rubbish.
We look forward to the release of System 03, which promises potentially capturing plastics at a rate 10x of System 002. At Dataiku, we are happy to play a part in bringing AI to solve this problem and in leaving the world a little better than we found it.