Autonomous AI Agents are revolutionizing the landscape of multiple industries by automating tasks and driving efficiencies. As AI continues to mature, various subsectors within the Autonomous Agent industry are emerging, offering distinctive value propositions.
Catch up on Part 1 of our Autonomous AI Agents article
In this article, we’ll break down the generalized workflow of an Autonomous AI Agent, using a Social Media Manager as an example. We’ll then dive into the subsectors that are emerging, particularly focusing on AgentOps—a field that is democratizing AI infrastructure and providing critical tools for AI engineers, ultimately enabling and expanding the very workflow we begin this article with.
Generalized framework for how autonomous AI agents work
Autonomous AI agents operate based on a set of defined steps that guide them in accomplishing specific goals. For clarity, let’s walk through an example featuring an autonomous social media manager agent.
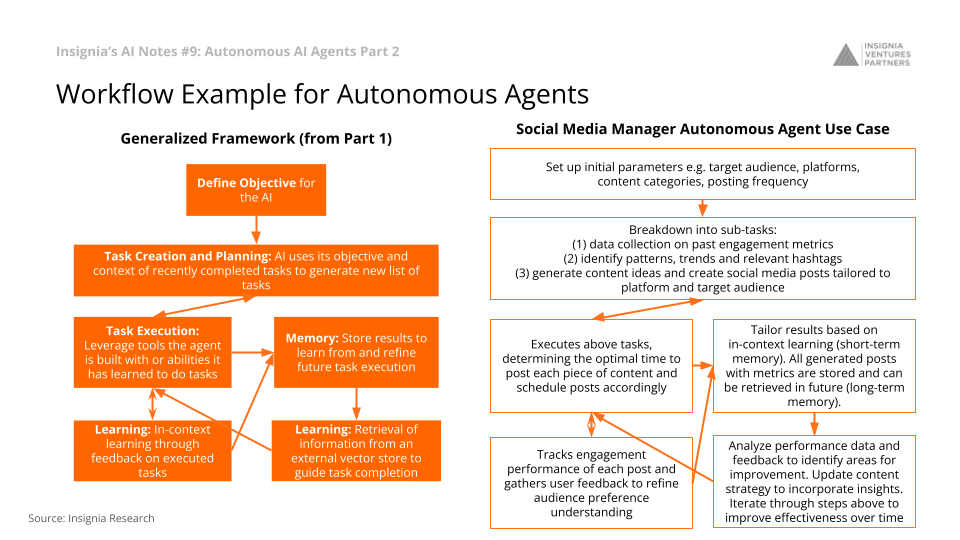
- Initialize goal: Define the objective for the AI. The Social Media Manager Agent will set up initial parameters like target audience, platforms, content categories, and posting frequency
- Task creation: Generate a list of tasks based on objectives and context. The Social Media Manager Agent will break down its objective into sub-tasks, such as data collection, pattern recognition, and content creation
- Task execution: Execute tasks autonomously or in conjunction with other agents, tools, or humans. The Social Media Manager Agent will determine optimal posting times and schedule content
- Feedback collection: Gather data to gauge the performance of tasks. The Social Media Manager Agent will collect metrics and user feedback
- Memory storage: Store results in a vector database. The Social Media Manager Agent will use both short-term and long-term memory storage
- Iteration: Learn from experiences and adapt based on new information. The Social Media Manager Agent will update strategies and improve effectiveness over time.
Autonomous AI agents subsector overview
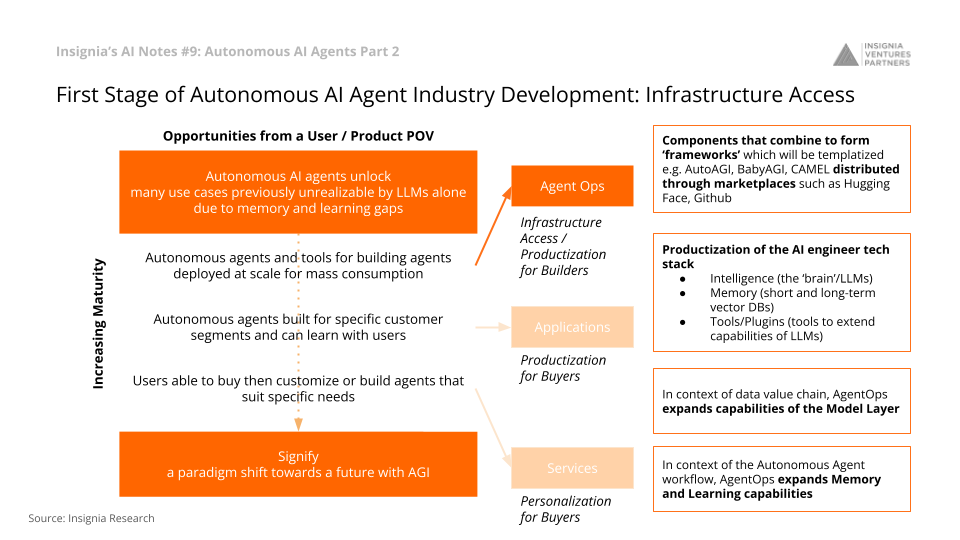
Emerging from the capabilities of Autonomous AI Agents are specialized subsectors designed to offer targeted solutions.
- AgentOps: Infrastructure and tools for builders, aimed at mass deployment
- Applications: Customer-segment specific agents with learning capabilities
- Services: Customizable agents for buyers who have unique needs
Deep dive into AgentOps: Enabling the autonomous AI agent workflow
AgentOps, or Agent Operations, is instrumental in building the foundation on which Autonomous AI Agents operate. These are often components (models, databases, and tools/plugins) that combine to form ‘frameworks’ that will be templatized e.g. AutoAGI, BabyAGI, CAMEL, and distributed through marketplaces such as Hugging Face, and Github. AgentOps hence primarily focuses on the productization of the AI engineer tech stack, which we can segment into three areas:
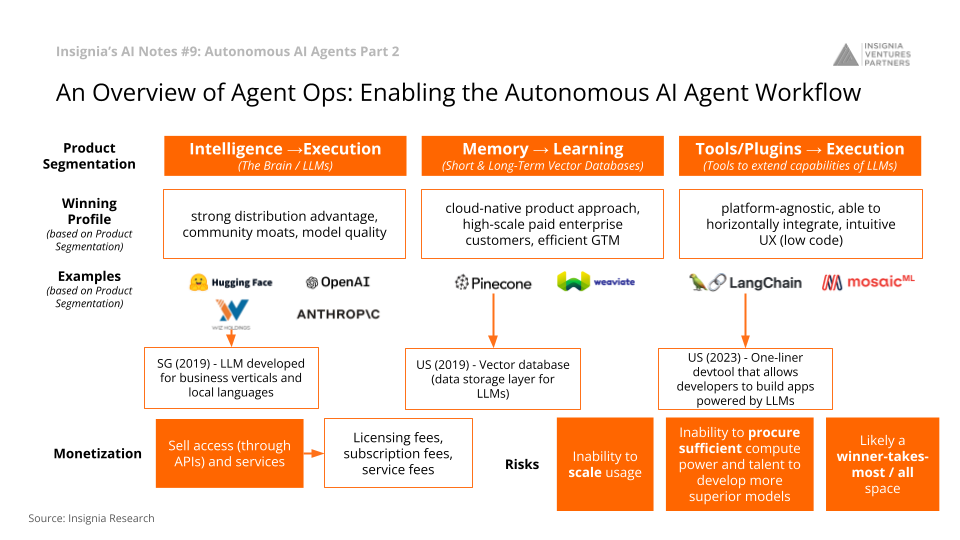
Intelligence
Intelligence is the brain of the Large Language Models on which agent execution is based. Learning can also leverage these models. Winners in this space would need to have a strong distribution advantage, community moats, and model quality. An example in this area is WIZ.AI (portfolio company), a voice AI company that has developed an LLM for business verticals and local languages.
Memory
Memory covers short and long-term vector databases. These databases are key for memory and hence learning as well when it comes to the autonomous agent workflow. Winners in this space would need to have a cloud-native product approach, high-scale paid enterprise customers, and efficient GTM. An example in this space is Pinecone from the US, which offers a vector database or data storage layer for LLMs.
Tools/plugins
These tools and plugins are third-party components leveraged by Autonomous AI Agent workflows often to supplement gaps in the core model being used given the scope of the objective, or to build up new workflows for specific use cases. These ultimately extend the capabilities of LLMs. Winners in this space would need to be platform-agnostic, able to horizontally integrate, and have intuitive UX for builders (i.e., low code). An example in this space is LangChain, a one-liner Devtool that allows developers to build apps powered by LLMs
Monetization and risks for AgentOps
Monetization strategies: Licensing fees, subscription-based models, and service fees are common.
Risks: This subsector faces challenges like resource scarcity, scalability issues, and fierce competition, which could lead to a ‘winner-takes-all’ scenario.
The advent of Autonomous AI Agents is opening doors to countless opportunities, but it’s the underlying infrastructure and subsectors like AgentOps that are laying the groundwork for sustainable growth and innovation. By understanding how these autonomous agents operate, and by dissecting the emerging subsectors, businesses and developers can better position themselves to leverage AI’s transformative power.
Stay tuned as we continue to explore the other subsectors that are making waves in the world of Autonomous AI Agents.
Follow our LinkedIn page for the latest updates on our weekly AI insights and other insights in Southeast Asia’s innovation landscape.
Author’s Note: This article was written with insights and research from our investment team. Reach out to them if you’re building in this space out of Southeast Asia and would like a deeper conversation.
Paulo Joquiño is a writer and content producer for tech companies, and co-author of the book Navigating ASEANnovation. He is currently Editor of Insignia Business Review, the official publication of Insignia Ventures Partners, and senior content strategist for the venture capital firm, where he started right after graduation. As a university student, he took up multiple work opportunities in content and marketing for startups in Asia. These included interning as an associate at G3 Partners, a Seoul-based marketing agency for tech startups, running tech community engagements at coworking space and business community, ASPACE Philippines, and interning at workspace marketplace FlySpaces. He graduated with a BS Management Engineering at Ateneo de Manila University in 2019.
This article is the first part of a series on AI and was originally published in the Insignia Business Review.
TNGlobal INSIDER publishes contributions relevant to entrepreneurship and innovation. You may submit your own original or published contributions subject to editorial discretion.